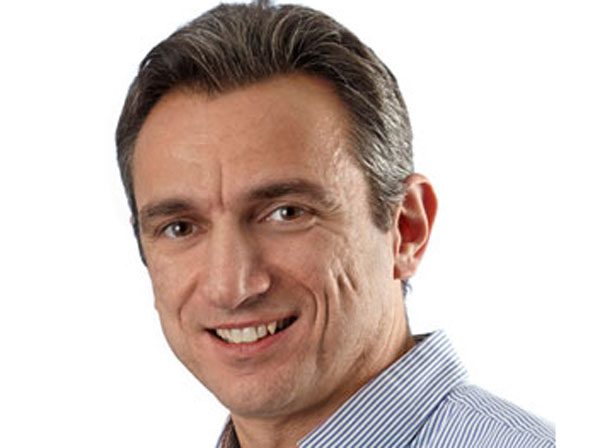
Click to learn more about author Babis Marmanis.
When the COVID-19 pandemic hit us, the global research community went into high gear to study the disease and to share their research in hopes of finding a solution. This increase in research output created a new challenge for scientific publishers: finding enough qualified peer-reviewers to keep up with the influx of manuscript submissions.
This blog is the continuation of a talk that I gave at the Outsell Signature Event in November 2020, where I participated in a panel discussion with CCC President and CEO Tracey Armstrong and moderator David Worlock on “Using AI to Create Collaboration, Partnership, and New Business Opportunities: Launching the CCC Knowledge Graph.”
There had been thousands of manuscripts submitted weekly since early in 2020, just in that area of research alone. From the perspective of a publisher overseeing peer-reviewed journals, that is a tremendous number of new manuscripts to vet, edit, and publish. The demand for quick turnaround of high-quality reviews, in order to accelerate progress, was further intensifying the pressure of identifying good candidates.
As everyone rushed to combat COVID, we also wanted to contribute what we could. By leveraging our data and technology, we developed a knowledge graph to help publishers address the problem of identifying suitable candidates for peer reviews in the COVID space. So, in what follows, I will describe in what sense our approach can be of significance beyond the COVID-specific work.
To begin with, we need to emphasize that the key term in “knowledge graph” is the word “knowledge” rather than “graph.” Hence, we should first define what the word “knowledge” means for our discussion. There is a long-standing debate about the data value chain as promoted by Russell Ackoff. The Data-Information-Knowledge-Wisdom (DIKW) hierarchy, as it came to be known, was brought to prominence by his address to the International Society for General Systems Research in 1989. At the highest level, it is generally accepted that the data value chain can be summarized by two key transitions:
1. A transition from “raw data” to “information,” and
2. A transition from “information” to “knowledge.”
Now, let us look at these terms more closely. We will define “information” as data that is fit for purpose within a specific context. For any set of data to be considered as “information,” a certain degree of data cleansing, data integration, and possibly data enrichment must take place.
With that in mind, let us now define “knowledge” as “actionable information.” It is important to note that knowledge must necessarily be associated with a degree of confidence that expresses the strength of our conviction about the accuracy of the information. Therefore, much like our own beliefs, it cannot be static. Our beliefs continuously evolve and adjust to accommodate new information, and, in turn, that results in adjustments of the confidence that we have about our knowledge.
True knowledge is not attainable. Take the field of physics, for example; the true nature of things is not possible to find. As Feynman put it: “We are never definitely right; we can only be sure we are wrong.” Yet, this hasn’t stopped us from creating very successful models of reality and using them to exert our control over nature in numerous ways.
Creating conceptual models based on data about our businesses will be essential for success in the 21st century, and a knowledge-based system is a great way of creating these conceptual models. Once you have a model, you can integrate it into your operational environment, measure its variables, observe its dynamics, incorporate operational measures based on different model criteria, and continuously refine and adjust it. In my opinion, that is where the true value of Data Science lies.
That’s something that any sensible person would agree with, and many people claim to have accomplished. I think that it is far from trivial to accomplish even if you narrow the scope of your knowledge-based system to a specific area of your business. Take, for example, the knowledge graph that I mentioned earlier.
Our graph relies on a dataset that consists of published scientific articles in virology with special attention to coronaviruses, including SARS, MERS, and SAR-CoV-2. We used bibliographic citation metadata for articles listed by LitCovid, CORD-19, and other sources. All in all, we processed over 120,000 articles.
Our thinking was fairly straightforward; if we can show the various authors, their associated literature, their collaborators (co-authors), and some general characterization of the field of their study, then a match between an arriving manuscript and an appropriate reviewer could be readily made. However, even with such a limited set of data, there are plenty of questions to answer and a significant degree of uncertainty to deal with.
Is “Ralph S Baric” of publication A the same author as “R S Baric” of publication B? And, how about that “Ralph A Baric” guy from publication C? Is he the same person, a cousin, a lexicographic coincidence, or simply an error? When we assign a MeSH term to an article, at what level of the MeSH hierarchy should we make the assignment? Should that depend on our level of confidence or be fixed a priori? Should we consider the full text in making our classification (if available) or use only bibliographic metadata? Should we provide the provenance of our beliefs or simply store the present state? How about the institution names? At what level should we capture the affiliation? If there is more than one affiliation, are any of them transient? Which one really matters for the purpose of contacting the author? I could go on and on with a list of questions that one needs to consider in order to arrive at a stage that the information in the system has achieved a level of confidence that allows us to make it actionable. The state of the data that raises these questions is directly tied to the information entropy in the system, and therefore, these questions multiply as the size of the system grows.
To address the above questions and many others, we processed the data through a specially crafted data pipeline in order to extract the appropriate metadata and disambiguate author names, author affiliations, and their publishing relationships to other authors. That process produced approximately 440,000 unique authors.
Although we are only visualizing that knowledge at the moment, we have built an extensible and open architecture that will allow the knowledge to be transfused into many other applications. One can’t help but think of what would be possible when our approach brings together more data from our customers, our partners, and even other third parties. Since a knowledge graph represents a belief system, there isn’t a single knowledge graph to rule them all!
Sure, there is a common denominator between any two knowledge graphs that are produced from the same data or to serve in the same field, but a large part of the business value is to be sought in their differences rather than their similarities. Our view is that building a knowledge graph system, essentially, means building a belief system for your business.
A system that can understand the intent of your users in various circumstances and provide the power of knowledge to employees, and end-users alike, at the right place and at the right time.
A living, breathing system that continuously evolves and absorbs new information and that is tightly coupled to the “organs” of your business, presenting the “truth” as your business perceives it.
In that way, data, content, and services become semantically interoperable, allowing AI agents to understand your business and perform tasks with great effectiveness. The time when people were browsing through a large number of documents, websites, and other sources of
content and manually extracting and interpreting the information within them is not the future. In fact, it is becoming increasingly the past. Users nowadays ask their personal assistants to perform knowledge-backed tasks without delving into the required process for that task themselves.
If you take nothing else away from this post, remember this:
- A knowledge graph is a great way to encapsulate the view of the world in the context of your business, i.e., your belief system
- A knowledge graph will continuously provide an ROI if it constantly evolves and incorporates new information that enables new uses
Businesses that do this will be able to expand further the reach of their services, improve the quality of their operations, and bring new products to many new customers. That is not an easy task, but it can be a very rewarding endeavor.