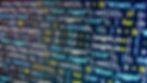
Data is the lifeblood of a business. When you have good data, your business will be able to make better decisions and see what’s working or not working with ease.
Every year, organizations suffer an average of USD 12.9 million due to poor data quality. Not only does the revenue get hit, but the long-term impact is also the complexity of data ecosystems and impaired decision-making. As per the predictions by Gartner, by 2022, 70% of organizations will thoroughly monitor data quality levels by applying metrics and thereby improving them by 60% and bringing down their operational risks and costs.
As companies grow and expand into new markets, the quality of their data is essential to predict potentials accurately.
Find out in this blog post which metrics successful companies use and how they can help you measure data quality in your own company!
Why should organizations measure data quality?
The value of high-quality data can be exemplified by looking at the big four. Google, Microsoft, Amazon, and Facebook reportedly store 1,200 petabytes. between them. It is not even close to the amount of data available on the internet. However, what’s also important is the quality of data because poor data quality is one costly affair.
Without quality data on hand, you will have ambiguity regarding customer needs. But, when you have a sense of their wants and preferences, you have a better way to reach out to them. Data obtained from purchase information or surveys are a couple of ways to know your customers.
However, suppose you fail to recognize the quality data. In that case, the organization will make poor decisions, launch ineffective marketing campaigns that don’t pander to the right audience, and eventually lead to attrition of customers or users.
1. Cost Optimization
As mentioned earlier, poor data quality is not favorable for any organization. Moreover, data collection incurs significant costs because of the time and effort. Imagine if all that effort went in vain. In fact, as per Gartner, organizations face an average financial impact of around USD 15 million per year. In comparison, another study found that poor data quality cost businesses at least 30% of revenues.
2. Effective Marketing
Good quality, accurate data is vital for marketers to decide who to market to and how. When the organization equips itself with such critical data, it knows what it’s doing and can launch effective marketing campaigns.
3. Better Decision-Making
An organization's ability to make quick decisions can help them mitigate risks. It is possible when there's good enough data to work. With the superior quality of data, top management and the organization can make decisions with lower risk possibilities.
4. Productivity
Analysts usually spend a lot of time scrutinizing and validating their data before it finds its use in strategic decision-making. It is why more automation can take place when a data management process churns out consistent, high-quality data. It translates to employees not being required to spend several hours making changes to data and rather focus on other areas.
5. Compliance
Many organizations have to adhere to specific regulations and responsibilities concerning compliance to store data. These compliance concerns are ongoing processes and need constant attention. And dashboard-type analytics are now an essential way for organizations, like those in the financial industry, to know whether or not they are keeping up with compliance standards.
7 Metrics to measure the quality of your data
1. The ratio of data to errors
A popular metric of measurement for data quality. You get to track how the number of known errors that occur within a data set (missing, incomplete or redundant entries) corresponds to the size of the data set. When the error count is low and the data size is the same, it’s a sign that your data quality is good.
2. Number of empty values
Empty values refer to the missing or recorded information in the wrong section. It is easy to track these errors within a data set. For example, you can track the number of empty fields within a data set and then change this number.
3. Data transformation error rates
When problems occur during data transformation, poor data quality often occurs. Data transformation is when stored data is taken in one format and converted into another format. And when you find there are several times the data transformation process fails, you will know about the quality of your data.
4. Amounts of dark data
The data you can't use because of quality issues is dark data. So when the organization has more dark data, it means there are a lot of quality issues in the data they are storing.
5. Email bounce rates
Poor data quality is a sign if you have higher email bounce rates. For example, imagine you’re running a marketing campaign, and you want to communicate about it to current and potential customers. But because of wrong information, errors, outdated data, you send emails to the wrong addresses. And this would not serve any purpose leading to a waste of time and effort.
6. Data storage costs
If you find that your data storage costs increase while the amount you use is the same, there are data quality issues. However, if your storage costs go down while the amount you use stays the same, that’s a sign that you’re doing reasonably well on the quality front.
7. Data time-to-value
Refers to the time taken by your team to derive results from the data. Although several reasons influence this, poor data quality is one of the most common factors that can pull down your team’s efforts to derive useful information from raw data.
In the end, the above is just the array of guidelines you can follow. The metrics you end up using will depend on the organization’s needs.
Wrapping up
Now that we know the importance of quality data and the impact of poor data quality, it's time for organizations to bring about a change in their way of dealing with data. Not only does poor data drain the organization financially, but it also brings down productivity.
At Datahut, we undertake various steps to provide clean, error-free, and ready-to-use data. It can help you minimize the number of issues during data conversions or in deriving faster results. In a world where data is the key to success, go for a reliable service provider like Datahut to provide you with all data-related solutions
Related Reading: 1. How to Create High-Quality Product Data Feeds?